--- license: apache-2.0 prior: - kandinsky-community/kandinsky-2-2-prior tags: - text-to-image - kandinsky inference: false --- # Kandinsky 2.2 Kandinsky inherits best practices from Dall-E 2 and Latent diffusion while introducing some new ideas. It uses the CLIP model as a text and image encoder, and diffusion image prior (mapping) between latent spaces of CLIP modalities. This approach increases the visual performance of the model and unveils new horizons in blending images and text-guided image manipulation. The Kandinsky model is created by [Arseniy Shakhmatov](https://github.com/cene555), [Anton Razzhigaev](https://github.com/razzant), [Aleksandr Nikolich](https://github.com/AlexWortega), [Igor Pavlov](https://github.com/boomb0om), [Andrey Kuznetsov](https://github.com/kuznetsoffandrey) and [Denis Dimitrov](https://github.com/denndimitrov) ## Usage Kandinsky 2.2 is available in diffusers! ```python pip install diffusers transformers accelerate ``` ### Text-to-Image Generation with ControlNet Conditioning ```python import torch import numpy as np from transformers import pipeline from diffusers.utils import load_image from diffusers import KandinskyV22PriorPipeline, KandinskyV22ControlnetPipeline # let's take an image and extract its depth map. def make_hint(image, depth_estimator): image = depth_estimator(image)["depth"] image = np.array(image) image = image[:, :, None] image = np.concatenate([image, image, image], axis=2) detected_map = torch.from_numpy(image).float() / 255.0 hint = detected_map.permute(2, 0, 1) return hint img = load_image( "https://huggingface.co/datasets/hf-internal-testing/diffusers-images/resolve/main/kandinskyv22/cat.png" ).resize((768, 768)) # We can use the `depth-estimation` pipeline from transformers to process the image and retrieve its depth map. depth_estimator = pipeline("depth-estimation") hint = make_hint(img, depth_estimator).unsqueeze(0).half().to("cuda") # Now, we load the prior pipeline and the text-to-image controlnet pipeline pipe_prior = KandinskyV22PriorPipeline.from_pretrained( "kandinsky-community/kandinsky-2-2-prior", torch_dtype=torch.float16 ) pipe_prior = pipe_prior.to("cuda") pipe = KandinskyV22ControlnetPipeline.from_pretrained( "kandinsky-community/kandinsky-2-2-controlnet-depth", torch_dtype=torch.float16 ) pipe = pipe.to("cuda") # We pass the prompt and negative prompt through the prior to generate image embeddings prompt = "A robot, 4k photo" negative_prior_prompt = "lowres, text, error, cropped, worst quality, low quality, jpeg artifacts, ugly, duplicate, morbid, mutilated, out of frame, extra fingers, mutated hands, poorly drawn hands, poorly drawn face, mutation, deformed, blurry, dehydrated, bad anatomy, bad proportions, extra limbs, cloned face, disfigured, gross proportions, malformed limbs, missing arms, missing legs, extra arms, extra legs, fused fingers, too many fingers, long neck, username, watermark, signature" generator = torch.Generator(device="cuda").manual_seed(43) image_emb, zero_image_emb = pipe_prior( prompt=prompt, negative_prompt=negative_prior_prompt, generator=generator ).to_tuple() # Now we can pass the image embeddings and the depth image we extracted to the controlnet pipeline. With Kandinsky 2.2, only prior pipelines accept `prompt` input. You do not need to pass the prompt to the controlnet pipeline. images = pipe( image_embeds=image_emb, negative_image_embeds=zero_image_emb, hint=hint, num_inference_steps=50, generator=generator, height=768, width=768, ).images images[0].save("robot_cat.png") ``` 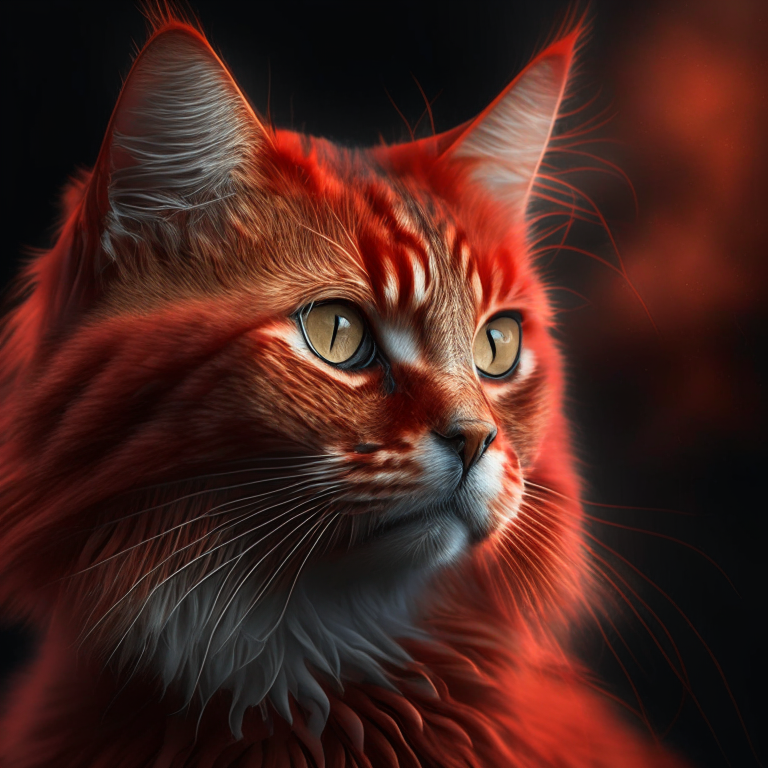 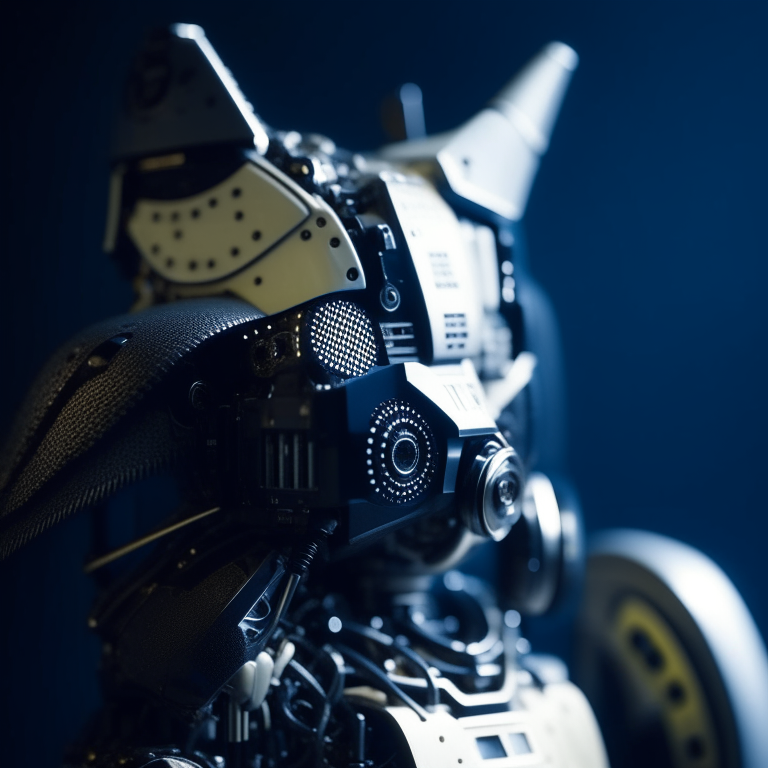 ### Image-to-Image Generation with ControlNet Conditioning ```python import torch import numpy as np from diffusers import KandinskyV22PriorEmb2EmbPipeline, KandinskyV22ControlnetImg2ImgPipeline from diffusers.utils import load_image from transformers import pipeline img = load_image( "https://huggingface.co/datasets/hf-internal-testing/diffusers-images/resolve/main" "/kandinskyv22/cat.png" ).resize((768, 768)) def make_hint(image, depth_estimator): image = depth_estimator(image)["depth"] image = np.array(image) image = image[:, :, None] image = np.concatenate([image, image, image], axis=2) detected_map = torch.from_numpy(image).float() / 255.0 hint = detected_map.permute(2, 0, 1) return hint depth_estimator = pipeline("depth-estimation") hint = make_hint(img, depth_estimator).unsqueeze(0).half().to("cuda") pipe_prior = KandinskyV22PriorEmb2EmbPipeline.from_pretrained( "kandinsky-community/kandinsky-2-2-prior", torch_dtype=torch.float16 ) pipe_prior = pipe_prior.to("cuda") pipe = KandinskyV22ControlnetImg2ImgPipeline.from_pretrained( "kandinsky-community/kandinsky-2-2-controlnet-depth", torch_dtype=torch.float16 ) pipe = pipe.to("cuda") prompt = "A robot, 4k photo" negative_prior_prompt = "lowres, text, error, cropped, worst quality, low quality, jpeg artifacts, ugly, duplicate, morbid, mutilated, out of frame, extra fingers, mutated hands, poorly drawn hands, poorly drawn face, mutation, deformed, blurry, dehydrated, bad anatomy, bad proportions, extra limbs, cloned face, disfigured, gross proportions, malformed limbs, missing arms, missing legs, extra arms, extra legs, fused fingers, too many fingers, long neck, username, watermark, signature" generator = torch.Generator(device="cuda").manual_seed(43) # run prior pipeline img_emb = pipe_prior(prompt=prompt, image=img, strength=0.85, generator=generator) negative_emb = pipe_prior(prompt=negative_prior_prompt, image=img, strength=1, generator=generator) # run controlnet img2img pipeline images = pipe( image=img, strength=0.5, image_embeds=img_emb.image_embeds, negative_image_embeds=negative_emb.image_embeds, hint=hint, num_inference_steps=50, generator=generator, height=768, width=768, ).images images[0].save("robot_cat.png") ``` Here is the output. Compared with the output from our text-to-image controlnet example, it kept a lot more cat facial details from the original image and worked into the robot style we asked for.  ## Model Architecture ### Overview Kandinsky 2.2 is a text-conditional diffusion model based on unCLIP and latent diffusion, composed of a transformer-based image prior model, a unet diffusion model, and a decoder. The model architectures are illustrated in the figure below - the chart on the left describes the process to train the image prior model, the figure in the center is the text-to-image generation process, and the figure on the right is image interpolation.