Ameer Azam
ameerazam08
AI & ML interests
Gen AI || Deep Learning || Transfer Learning
Recent Activity
new activity
2 days ago
ameerazam08/Diffusion-Eraser:EXEC env Variable = Closed Source
Organizations
ameerazam08's activity
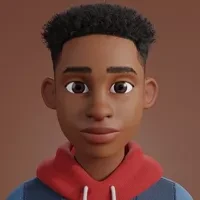
reacted to
reach-vb's
post with 👍
2 months ago
Post
4572
Massive week for Open AI/ ML:
Mistral Pixtral & Instruct Large - ~123B, 128K context, multilingual, json + function calling & open weights
mistralai/Pixtral-Large-Instruct-2411
mistralai/Mistral-Large-Instruct-2411
Allen AI Tülu 70B & 8B - competive with claude 3.5 haiku, beats all major open models like llama 3.1 70B, qwen 2.5 and nemotron
allenai/tulu-3-models-673b8e0dc3512e30e7dc54f5
allenai/tulu-3-datasets-673b8df14442393f7213f372
Llava o1 - vlm capable of spontaneous, systematic reasoning, similar to GPT-o1, 11B model outperforms gemini-1.5-pro, gpt-4o-mini, and llama-3.2-90B-vision
Xkev/Llama-3.2V-11B-cot
Black Forest Labs Flux.1 tools - four new state of the art model checkpoints & 2 adapters for fill, depth, canny & redux, open weights
reach-vb/black-forest-labs-flux1-6743847bde9997dd26609817
Jina AI Jina CLIP v2 - general purpose multilingual and multimodal (text & image) embedding model, 900M params, 512 x 512 resolution, matroyoshka representations (1024 to 64)
jinaai/jina-clip-v2
Apple AIM v2 & CoreML MobileCLIP - large scale vision encoders outperform CLIP and SigLIP. CoreML optimised MobileCLIP models
apple/aimv2-6720fe1558d94c7805f7688c
apple/coreml-mobileclip
A lot more got released like, OpenScholar (https://huggingface.co/collections/OpenScholar/openscholar-v1-67376a89f6a80f448da411a6), smoltalk ( HuggingFaceTB/smoltalk), Hymba ( nvidia/hymba-673c35516c12c4b98b5e845f), Open ASR Leaderboard ( hf-audio/open_asr_leaderboard) and much more..
Can't wait for the next week! 🤗
Mistral Pixtral & Instruct Large - ~123B, 128K context, multilingual, json + function calling & open weights
mistralai/Pixtral-Large-Instruct-2411
mistralai/Mistral-Large-Instruct-2411
Allen AI Tülu 70B & 8B - competive with claude 3.5 haiku, beats all major open models like llama 3.1 70B, qwen 2.5 and nemotron
allenai/tulu-3-models-673b8e0dc3512e30e7dc54f5
allenai/tulu-3-datasets-673b8df14442393f7213f372
Llava o1 - vlm capable of spontaneous, systematic reasoning, similar to GPT-o1, 11B model outperforms gemini-1.5-pro, gpt-4o-mini, and llama-3.2-90B-vision
Xkev/Llama-3.2V-11B-cot
Black Forest Labs Flux.1 tools - four new state of the art model checkpoints & 2 adapters for fill, depth, canny & redux, open weights
reach-vb/black-forest-labs-flux1-6743847bde9997dd26609817
Jina AI Jina CLIP v2 - general purpose multilingual and multimodal (text & image) embedding model, 900M params, 512 x 512 resolution, matroyoshka representations (1024 to 64)
jinaai/jina-clip-v2
Apple AIM v2 & CoreML MobileCLIP - large scale vision encoders outperform CLIP and SigLIP. CoreML optimised MobileCLIP models
apple/aimv2-6720fe1558d94c7805f7688c
apple/coreml-mobileclip
A lot more got released like, OpenScholar (https://huggingface.co/collections/OpenScholar/openscholar-v1-67376a89f6a80f448da411a6), smoltalk ( HuggingFaceTB/smoltalk), Hymba ( nvidia/hymba-673c35516c12c4b98b5e845f), Open ASR Leaderboard ( hf-audio/open_asr_leaderboard) and much more..
Can't wait for the next week! 🤗
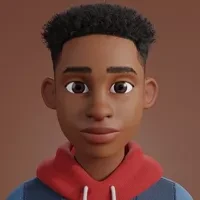
reacted to
davidberenstein1957's
post with 🚀
2 months ago
Post
3451
The Data Is Better Together community is set to release the first Apache 2 licensed image preference dataset!
Great work and let's give this a final push :)
@aashish1904 congrats on your month of HF pro. There is more to win during this sprint!
@aashish1904 @AnyaDesdein @davidberenstein1957 @Malalatiana @beta3 @fffiloni @munish0838 @Reza2kn @bbunzeck @Creazycreator @andrei-saceleanu @jafhaponiuk @rca-etl @kf120 @burtenshaw @mmhamdy @grib0ed0v @Doopus @AnyaDes @ttkap @Xceron @Lewox @davanstrien @Azazelle @adirik @Ashish08 @AntonVic @kenantang @sdiazlor @g-ronimo @dennis-rall @prithivMLmods @girtss3 @flozi00 @WaveCut @Taylor658 @Wildminder @Sara9999 @phaelishall @sararob @dvilasuero @pgabrys @plaguss @CDS899 @timajwilliams @rudzinskimaciej @pavel-ai @aggr8 @ignacioct @MouseAI @Leeps @MaksKul @NicolasDmln @Muinez @kusht55 @caiolang @Jakub-Brand24 @loamy @Demijan @eliab96 @Viewegger @JosephCatrambone @p1atdev @mrshu @o639 @Targezed @Aviv-anthonnyolime @thliang01 @Ahmed-Amine @glards @pranaykoppula @nataliaElv @MaPirlet @alvarobartt @gabrielmbmb @zlicastro @Jaydip @Chouettecheveche @lilcheaty @ruyrdiaz @robintema @fdaudens @ggcristian @a-r-r-o-w @pates @joheras @stopsatgreen @bezo97 @chachi902 @iamyann @liamcripwell @dmb23 @korbih @anonymous7743 @akbdx18 @OVAWARE @severo @akontra @lichorosario @lhoestq @SebastianBodza @Vishnou @ameerazam08 @appoose @Mukei @mearco @joaquincabezas @Fizzarolli @thomastraum @igortopolski @OxxoCodes @patrickfleith @asoria @bn22 @sitammeur @Krodolf @bergr7f @Sbxxn @wietsevenema @sugatoray @Iamladi @MikeTrizna @feveromo @mokady @Bolero @prath @Dowwie @kfahn @decodingchris @alili2050 @RahulRaman @yzimmermann @Ameeeee @ecyht2 @MattMC001 @hemanthkumarak @Thegorgibus @akos2 @LawRun @ramithuh @SuperMuel @sjans @peterizsak @mosama @Eyel @mtr3 @cfahlgren1 @legentil @clem @Citaman @Aurelien-Morgan @AntoineBourgois @TotoB12 @Stanmey @osanseviero @multimodalart @maxiw @ariG23498 @ngk89 @femboysLover @dvs @tacohiddink @blanchon @DavidJimenez
Great work and let's give this a final push :)
@aashish1904 congrats on your month of HF pro. There is more to win during this sprint!
@aashish1904 @AnyaDesdein @davidberenstein1957 @Malalatiana @beta3 @fffiloni @munish0838 @Reza2kn @bbunzeck @Creazycreator @andrei-saceleanu @jafhaponiuk @rca-etl @kf120 @burtenshaw @mmhamdy @grib0ed0v @Doopus @AnyaDes @ttkap @Xceron @Lewox @davanstrien @Azazelle @adirik @Ashish08 @AntonVic @kenantang @sdiazlor @g-ronimo @dennis-rall @prithivMLmods @girtss3 @flozi00 @WaveCut @Taylor658 @Wildminder @Sara9999 @phaelishall @sararob @dvilasuero @pgabrys @plaguss @CDS899 @timajwilliams @rudzinskimaciej @pavel-ai @aggr8 @ignacioct @MouseAI @Leeps @MaksKul @NicolasDmln @Muinez @kusht55 @caiolang @Jakub-Brand24 @loamy @Demijan @eliab96 @Viewegger @JosephCatrambone @p1atdev @mrshu @o639 @Targezed @Aviv-anthonnyolime @thliang01 @Ahmed-Amine @glards @pranaykoppula @nataliaElv @MaPirlet @alvarobartt @gabrielmbmb @zlicastro @Jaydip @Chouettecheveche @lilcheaty @ruyrdiaz @robintema @fdaudens @ggcristian @a-r-r-o-w @pates @joheras @stopsatgreen @bezo97 @chachi902 @iamyann @liamcripwell @dmb23 @korbih @anonymous7743 @akbdx18 @OVAWARE @severo @akontra @lichorosario @lhoestq @SebastianBodza @Vishnou @ameerazam08 @appoose @Mukei @mearco @joaquincabezas @Fizzarolli @thomastraum @igortopolski @OxxoCodes @patrickfleith @asoria @bn22 @sitammeur @Krodolf @bergr7f @Sbxxn @wietsevenema @sugatoray @Iamladi @MikeTrizna @feveromo @mokady @Bolero @prath @Dowwie @kfahn @decodingchris @alili2050 @RahulRaman @yzimmermann @Ameeeee @ecyht2 @MattMC001 @hemanthkumarak @Thegorgibus @akos2 @LawRun @ramithuh @SuperMuel @sjans @peterizsak @mosama @Eyel @mtr3 @cfahlgren1 @legentil @clem @Citaman @Aurelien-Morgan @AntoineBourgois @TotoB12 @Stanmey @osanseviero @multimodalart @maxiw @ariG23498 @ngk89 @femboysLover @dvs @tacohiddink @blanchon @DavidJimenez
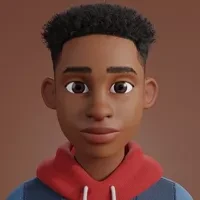
reacted to
DmitryRyumin's
post with 🔥
7 months ago
Post
3673
🚀🎭🌟 New Research Alert - Portrait4D-v2 (Avatars Collection)! 🌟🎭🚀
📄 Title: Portrait4D-v2: Pseudo Multi-View Data Creates Better 4D Head Synthesizer 🔝
📝 Description: Portrait4D-v2 is a novel method for one-shot 4D head avatar synthesis using pseudo multi-view videos and a vision transformer backbone, achieving superior performance without relying on 3DMM reconstruction.
👥 Authors: Yu Deng, Duomin Wang, and Baoyuan Wang
📄 Paper: Portrait4D-v2: Pseudo Multi-View Data Creates Better 4D Head Synthesizer (2403.13570)
🌐 GitHub Page: https://yudeng.github.io/Portrait4D-v2/
📁 Repository: https://github.com/YuDeng/Portrait-4D
📺 Video: https://www.youtube.com/watch?v=5YJY6-wcOJo
🚀 CVPR-2023-24-Papers: https://github.com/DmitryRyumin/CVPR-2023-24-Papers
📚 More Papers: more cutting-edge research presented at other conferences in the DmitryRyumin/NewEraAI-Papers curated by @DmitryRyumin
🚀 Added to the Avatars Collection: DmitryRyumin/avatars-65df37cdf81fec13d4dbac36
🔍 Keywords: Portrait4D #4DAvatar #HeadSynthesis #3DModeling #TechInnovation #DeepLearning #ComputerGraphics #ComputerVision #Innovation
📄 Title: Portrait4D-v2: Pseudo Multi-View Data Creates Better 4D Head Synthesizer 🔝
📝 Description: Portrait4D-v2 is a novel method for one-shot 4D head avatar synthesis using pseudo multi-view videos and a vision transformer backbone, achieving superior performance without relying on 3DMM reconstruction.
👥 Authors: Yu Deng, Duomin Wang, and Baoyuan Wang
📄 Paper: Portrait4D-v2: Pseudo Multi-View Data Creates Better 4D Head Synthesizer (2403.13570)
🌐 GitHub Page: https://yudeng.github.io/Portrait4D-v2/
📁 Repository: https://github.com/YuDeng/Portrait-4D
📺 Video: https://www.youtube.com/watch?v=5YJY6-wcOJo
🚀 CVPR-2023-24-Papers: https://github.com/DmitryRyumin/CVPR-2023-24-Papers
📚 More Papers: more cutting-edge research presented at other conferences in the DmitryRyumin/NewEraAI-Papers curated by @DmitryRyumin
🚀 Added to the Avatars Collection: DmitryRyumin/avatars-65df37cdf81fec13d4dbac36
🔍 Keywords: Portrait4D #4DAvatar #HeadSynthesis #3DModeling #TechInnovation #DeepLearning #ComputerGraphics #ComputerVision #Innovation
Post
4629
Explore the Latest Top Papers with Papers Leaderboard!
We are excited to introduce a new way to explore the most impactful research papers: Papers Leaderboard! This feature allows you to easily find the most talked-about papers across a variety of fields.
Hf-demo : ameerazam08/Paper-LeaderBoard
Happy weekends!
We are excited to introduce a new way to explore the most impactful research papers: Papers Leaderboard! This feature allows you to easily find the most talked-about papers across a variety of fields.
Hf-demo : ameerazam08/Paper-LeaderBoard
Happy weekends!
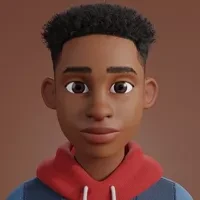
posted
an
update
10 months ago
Post
4629
Explore the Latest Top Papers with Papers Leaderboard!
We are excited to introduce a new way to explore the most impactful research papers: Papers Leaderboard! This feature allows you to easily find the most talked-about papers across a variety of fields.
Hf-demo : ameerazam08/Paper-LeaderBoard
Happy weekends!
We are excited to introduce a new way to explore the most impactful research papers: Papers Leaderboard! This feature allows you to easily find the most talked-about papers across a variety of fields.
Hf-demo : ameerazam08/Paper-LeaderBoard
Happy weekends!
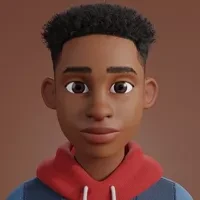
reacted to
DmitryRyumin's
post with 🔥
10 months ago
Post
3087
🚀💇♂️🔥 New Research Alert (Avatars Collection)! 🔥💇♀️🚀
📄 Title: HairFastGAN: Realistic and Robust Hair Transfer with a Fast Encoder-Based Approach
📝 Description: HairFastGAN is a fast, encoder-based approach to realistic and robust hair transfer that operates in the FS latent space of StyleGAN and includes enhanced in-painting and improved encoders for better alignment, color transfer, and post-processing.
👥 Authors: Maxim Nikolaev, Mikhail Kuznetsov, Dmitry Vetrov, and Aibek Alanov
🔗 Paper: HairFastGAN: Realistic and Robust Hair Transfer with a Fast Encoder-Based Approach (2404.01094)
📁 Repository: https://github.com/AIRI-Institute/HairFastGAN
🤗 Demo: multimodalart/hairfastgan
🔥 Model 🤖: AIRI-Institute/HairFastGAN
📚 More Papers: more cutting-edge research presented at other conferences in the DmitryRyumin/NewEraAI-Papers curated by @DmitryRyumin
🚀 Added to the Avatars Collection: DmitryRyumin/avatars-65df37cdf81fec13d4dbac36
🔍 Keywords: #HairFastGAN #StyleGAN #VirtualTryOn #HairTransfer #AIHairStyling #GenerativeModels #ComputerVision #ImageProcessing #DeepLearning
📄 Title: HairFastGAN: Realistic and Robust Hair Transfer with a Fast Encoder-Based Approach
📝 Description: HairFastGAN is a fast, encoder-based approach to realistic and robust hair transfer that operates in the FS latent space of StyleGAN and includes enhanced in-painting and improved encoders for better alignment, color transfer, and post-processing.
👥 Authors: Maxim Nikolaev, Mikhail Kuznetsov, Dmitry Vetrov, and Aibek Alanov
🔗 Paper: HairFastGAN: Realistic and Robust Hair Transfer with a Fast Encoder-Based Approach (2404.01094)
📁 Repository: https://github.com/AIRI-Institute/HairFastGAN
🤗 Demo: multimodalart/hairfastgan
🔥 Model 🤖: AIRI-Institute/HairFastGAN
📚 More Papers: more cutting-edge research presented at other conferences in the DmitryRyumin/NewEraAI-Papers curated by @DmitryRyumin
🚀 Added to the Avatars Collection: DmitryRyumin/avatars-65df37cdf81fec13d4dbac36
🔍 Keywords: #HairFastGAN #StyleGAN #VirtualTryOn #HairTransfer #AIHairStyling #GenerativeModels #ComputerVision #ImageProcessing #DeepLearning
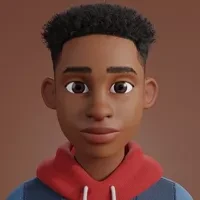
reacted to
abidlabs's
post with 👍
10 months ago
Post
3628
Open Models vs. Closed APIs for Software Engineers
-----------------------------------------------------------------------
If you're an ML researcher / scientist, you probably don't need much convincing to use open models instead of closed APIs -- open models give you reproducibility and let you deeply investigate the model's behavior.
But what if you are a software engineer building products on top of LLMs? I'd argue that open models are a much better option even if you are using them as APIs. For at least 3 reasons:
1) The most obvious reason is reliability of your product. Relying on a closed API means that your product has a single point-of-failure. On the other hand, there are at least 7 different API providers that offer Llama3 70B already. As well as libraries that abstract on top of these API providers so that you can make a single request that goes to different API providers depending on availability / latency.
2) Another benefit is eventual consistency going local. If your product takes off, it will be more economical and lower latency to have a dedicated inference endpoint running on your VPC than to call external APIs. If you've started with an open-source model, you can always deploy the same model locally. You don't need to modify prompts or change any surrounding logic to get consistent behavior. Minimize your technical debt from the beginning.
3) Finally, open models give you much more flexibility. Even if you keep using APIs, you might want to tradeoff latency vs. cost, or use APIs that support batches of inputs, etc. Because different API providers have different infrastructure, you can use the API provider that makes the most sense for your product -- or you can even use multiple API providers for different users (free vs. paid) or different parts of your product (priority features vs. nice-to-haves)
-----------------------------------------------------------------------
If you're an ML researcher / scientist, you probably don't need much convincing to use open models instead of closed APIs -- open models give you reproducibility and let you deeply investigate the model's behavior.
But what if you are a software engineer building products on top of LLMs? I'd argue that open models are a much better option even if you are using them as APIs. For at least 3 reasons:
1) The most obvious reason is reliability of your product. Relying on a closed API means that your product has a single point-of-failure. On the other hand, there are at least 7 different API providers that offer Llama3 70B already. As well as libraries that abstract on top of these API providers so that you can make a single request that goes to different API providers depending on availability / latency.
2) Another benefit is eventual consistency going local. If your product takes off, it will be more economical and lower latency to have a dedicated inference endpoint running on your VPC than to call external APIs. If you've started with an open-source model, you can always deploy the same model locally. You don't need to modify prompts or change any surrounding logic to get consistent behavior. Minimize your technical debt from the beginning.
3) Finally, open models give you much more flexibility. Even if you keep using APIs, you might want to tradeoff latency vs. cost, or use APIs that support batches of inputs, etc. Because different API providers have different infrastructure, you can use the API provider that makes the most sense for your product -- or you can even use multiple API providers for different users (free vs. paid) or different parts of your product (priority features vs. nice-to-haves)
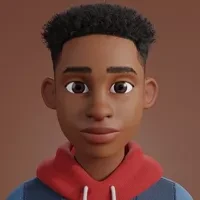
reacted to
Jaward's
post with 🔥
10 months ago
Post
3481
# On Coding Your First Attention
While it’s not necessarily the case that you must code the attention block of a transformer from scratch to understand how it works, yet it sure is the closest you can get to having a first-principles understanding of why/how transformers behave the way they do.
@karpathy covered attention in detail in his nanoGPT video (strongly recommend watching). Now I would like to share some thoughts and experience in writing my first attention.
First let’s zoom out quickly and explain what attention is in transformers: Attention in transformers is a communication mechanism that allows the model to focus on different parts of the input sequence when making predictions.
It assigns weights to each input token based on its relevance to the current context, enabling the model to weigh information selectively. This mechanism helps transformers capture long-range dependencies and contextual information effectively.
The official AIAN paper introduced two commonly used forms of attentions: Scaled Dot-Product Attention (also known as Self-Attention) and a stack of self-attention blocks known as Multi-Head Attention.
# The Code
Now, attention as for most deep learning algorithms boils down to a math equation. So writing the code can get really trivial especially with a deep learning framework like PyTorch. Below is what's called a Single Head Attention
(image 2)
The code defines single-head attention in PyTorch - it transforms input vectors, computes attention scores and weights, and then calculates the weighted sum of values based on these weights (as per the attention equation)
When you have multiple of those stacked in parallel, you get what's called Multi-Head Attention. This gives a much simpler code if you are inheriting from the SingleHeadAttention class:
(image 3)
Full Article here: https://huggingface.co/blog/Jaward/coding-your-first-attention
While it’s not necessarily the case that you must code the attention block of a transformer from scratch to understand how it works, yet it sure is the closest you can get to having a first-principles understanding of why/how transformers behave the way they do.
@karpathy covered attention in detail in his nanoGPT video (strongly recommend watching). Now I would like to share some thoughts and experience in writing my first attention.
First let’s zoom out quickly and explain what attention is in transformers: Attention in transformers is a communication mechanism that allows the model to focus on different parts of the input sequence when making predictions.
It assigns weights to each input token based on its relevance to the current context, enabling the model to weigh information selectively. This mechanism helps transformers capture long-range dependencies and contextual information effectively.
The official AIAN paper introduced two commonly used forms of attentions: Scaled Dot-Product Attention (also known as Self-Attention) and a stack of self-attention blocks known as Multi-Head Attention.
# The Code
Now, attention as for most deep learning algorithms boils down to a math equation. So writing the code can get really trivial especially with a deep learning framework like PyTorch. Below is what's called a Single Head Attention
(image 2)
The code defines single-head attention in PyTorch - it transforms input vectors, computes attention scores and weights, and then calculates the weighted sum of values based on these weights (as per the attention equation)
When you have multiple of those stacked in parallel, you get what's called Multi-Head Attention. This gives a much simpler code if you are inheriting from the SingleHeadAttention class:
(image 3)
Full Article here: https://huggingface.co/blog/Jaward/coding-your-first-attention
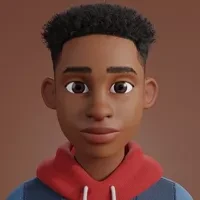
reacted to
MonsterMMORPG's
post with 🔥
11 months ago
Post
2006
Compared Effect Of Image Captioning For SDXL Fine-tuning / DreamBooth Training for a Single Person, 10.3 GB VRAM via OneTrainer
Sadly the post character count is limited so please read full info on Medium here
https://medium.com/@furkangozukara/compared-effect-of-image-captioning-for-sdxl-fine-tuning-dreambooth-training-for-a-single-person-961087e42334
Sadly the post character count is limited so please read full info on Medium here
https://medium.com/@furkangozukara/compared-effect-of-image-captioning-for-sdxl-fine-tuning-dreambooth-training-for-a-single-person-961087e42334
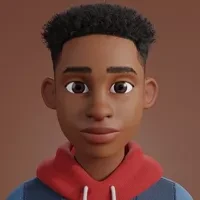
reacted to
MonsterMMORPG's
post with 👍
11 months ago
Post
I have dedicated several days, working over 12 hours each day, on SUPIR (Scaling-UP Image Restoration), a cutting-edge image enhancement and upscaling model introduced in the paper Scaling Up to Excellence: Practicing Model Scaling for Photo-Realistic Image Restoration In the Wild.
This model is simply mind-blowing. At the bottom of this post, you will see side-by-side comparisons of SUPIR versus the extremely expensive online service, Magnific AI. Magnific is known to be the best among the community. However, SUPIR is by far superior. SUPIR also significantly outperforms Topaz AI upscale. SUPIR manages to remain faithful to the original image almost 100% while adding details and achieving super upscaling with the best realism.
You can read the full blog post here : https://huggingface.co/blog/MonsterMMORPG/supir-sota-image-upscale-better-than-magnific-ai
This model is simply mind-blowing. At the bottom of this post, you will see side-by-side comparisons of SUPIR versus the extremely expensive online service, Magnific AI. Magnific is known to be the best among the community. However, SUPIR is by far superior. SUPIR also significantly outperforms Topaz AI upscale. SUPIR manages to remain faithful to the original image almost 100% while adding details and achieving super upscaling with the best realism.
You can read the full blog post here : https://huggingface.co/blog/MonsterMMORPG/supir-sota-image-upscale-better-than-magnific-ai
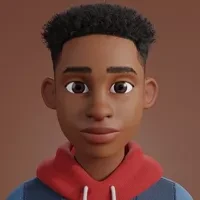
replied to
MonsterMMORPG's
post
11 months ago
This is Amzing Just 12GB
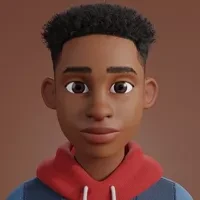
reacted to
mrfakename's
post with ❤️👍
11 months ago
Post
Today, I’m thrilled to release a project I’ve been working on for the past couple weeks in collaboration with Hugging Face: the TTS Arena.
The TTS Arena, inspired by LMSys's Chatbot Arena, allows you to enter text which will be synthesized by two SOTA models. You can then vote on which model generated a better sample. The results will be published on a publicly-accessible leaderboard.
We’ve added several open access models, including Pheme, MetaVoice, XTTS, OpenVoice, & WhisperSpeech. It also includes the proprietary ElevenLabs model.
If you have any questions, suggestions, or feedback, please don’t hesitate to DM me on X (https://twitter.com/realmrfakename) or open a discussion in the Space. More details coming soon!
Try it out: TTS-AGI/TTS-Arena
The TTS Arena, inspired by LMSys's Chatbot Arena, allows you to enter text which will be synthesized by two SOTA models. You can then vote on which model generated a better sample. The results will be published on a publicly-accessible leaderboard.
We’ve added several open access models, including Pheme, MetaVoice, XTTS, OpenVoice, & WhisperSpeech. It also includes the proprietary ElevenLabs model.
If you have any questions, suggestions, or feedback, please don’t hesitate to DM me on X (https://twitter.com/realmrfakename) or open a discussion in the Space. More details coming soon!
Try it out: TTS-AGI/TTS-Arena
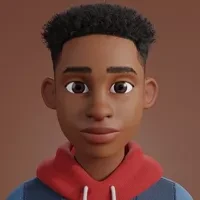
reacted to
s3nh's
post with 🤗👍
about 1 year ago
Post
GPU Poor POV: Dont be Afraid :D
Sometimes we dont want to do something because of low self esteem,
I ofter hear 'its to hard for me','i am not an expert','i do not know how to do it', etc. These words are never the truth, we should not be afraid and try to build something because there is no additive value without a failure.
Same things comes in LLMs, there is a lot of fancy words happening, but whats is more important is that there are also people who are constantly building so other can build. Diving into finetuning LLMs is incredibly simple if we assume using axolotl library and pretrains stored on huggingface.
All we need is an idea, our GPU Poor desktop or colab notebooks and these steps:
After installation process we can go to examples, and modify configs to our own needs.
Lets jump into
and change
to
choose dataset from huge amounts of dataset that are possible to use from hf.co/datasets and tweak additional params like batch_size, number of epochs, how often do we want to save our model and many more (which I wont focus on rn).
Then,
Will allow to start the finetuning process on structure defined strictly by you. After finetuning, model will be saved in path provided in config, and you can check out if it performs better than the base one. Or even you can put it on llm Leaderboard to check if we do not have new SOTA :)
Have fun and have a great day <3
Sometimes we dont want to do something because of low self esteem,
I ofter hear 'its to hard for me','i am not an expert','i do not know how to do it', etc. These words are never the truth, we should not be afraid and try to build something because there is no additive value without a failure.
Same things comes in LLMs, there is a lot of fancy words happening, but whats is more important is that there are also people who are constantly building so other can build. Diving into finetuning LLMs is incredibly simple if we assume using axolotl library and pretrains stored on huggingface.
All we need is an idea, our GPU Poor desktop or colab notebooks and these steps:
git clone https://github.com/OpenAccess-AI-Collective/axolotl
cd axolotl
pip3 install packaging
pip3 install -e '.[flash-attn,deepspeed]'
After installation process we can go to examples, and modify configs to our own needs.
Lets jump into
axolotl\examples\llama-2\qlora.yml
and change
base_model: NousResearch/Llama-2-7b-hf
to
base_model: TinyLlama/TinyLlama-1.1B-Chat-v1.0
choose dataset from huge amounts of dataset that are possible to use from hf.co/datasets and tweak additional params like batch_size, number of epochs, how often do we want to save our model and many more (which I wont focus on rn).
Then,
accelerate launch -m axolotl.cli.train examples/llama-2/qlora.yml
Will allow to start the finetuning process on structure defined strictly by you. After finetuning, model will be saved in path provided in config, and you can check out if it performs better than the base one. Or even you can put it on llm Leaderboard to check if we do not have new SOTA :)
Have fun and have a great day <3
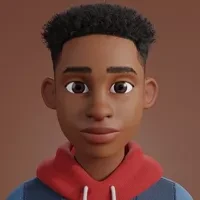
reacted to
clem's
post with 👍❤️
about 1 year ago
Post
Is synthetic data the future of AI? 🔥🔥🔥
@HugoLaurencon @Leyo & @VictorSanh are introducing HuggingFaceM4/WebSight , a multimodal dataset featuring 823,000 pairs of synthetically generated HTML/CSS codes along with screenshots of the corresponding rendered websites to train GPT4-V-like models 🌐💻
While crafting their upcoming foundation vision language model, they faced the challenge of converting website screenshots into usable HTML/CSS codes. Most VLMs suck at this and there was no public dataset available for this specific task, so they decided to create their own.
They prompted existing LLMs to generate 823k HTML/CSS codes of very simple websites. Through supervised fine-tuning of a vision language model on WebSight, they were able to generate the code to reproduce a website component, given a screenshot.
You can explore the dataset here: HuggingFaceM4/WebSight
What do you think?
@HugoLaurencon @Leyo & @VictorSanh are introducing HuggingFaceM4/WebSight , a multimodal dataset featuring 823,000 pairs of synthetically generated HTML/CSS codes along with screenshots of the corresponding rendered websites to train GPT4-V-like models 🌐💻
While crafting their upcoming foundation vision language model, they faced the challenge of converting website screenshots into usable HTML/CSS codes. Most VLMs suck at this and there was no public dataset available for this specific task, so they decided to create their own.
They prompted existing LLMs to generate 823k HTML/CSS codes of very simple websites. Through supervised fine-tuning of a vision language model on WebSight, they were able to generate the code to reproduce a website component, given a screenshot.
You can explore the dataset here: HuggingFaceM4/WebSight
What do you think?