Update README.md
Browse files
README.md
CHANGED
@@ -1,199 +1,88 @@
|
|
1 |
---
|
2 |
-
|
3 |
-
|
|
|
|
|
|
|
|
|
|
|
|
|
|
|
|
|
|
|
4 |
---
|
5 |
|
6 |
-
# Model Card for Model ID
|
7 |
|
8 |
-
|
9 |
-
|
10 |
-
|
11 |
-
|
12 |
-
|
13 |
-
|
14 |
-
|
15 |
-
|
16 |
-
|
17 |
-
|
18 |
-
|
19 |
-
|
20 |
-
|
21 |
-
|
22 |
-
|
23 |
-
- **
|
24 |
-
- **
|
25 |
-
-
|
26 |
-
-
|
27 |
-
|
28 |
-
|
29 |
-
|
30 |
-
|
31 |
-
|
32 |
-
|
33 |
-
|
34 |
-
|
35 |
-
|
36 |
-
|
37 |
-
|
38 |
-
|
39 |
-
|
40 |
-
|
41 |
-
|
42 |
-
|
43 |
-
|
44 |
-
|
45 |
-
|
46 |
-
|
47 |
-
|
48 |
-
|
49 |
-
|
50 |
-
|
51 |
-
|
52 |
-
|
53 |
-
|
54 |
-
|
55 |
-
|
56 |
-
|
57 |
-
|
58 |
-
|
59 |
-
|
60 |
-
|
61 |
-
|
62 |
-
|
63 |
-
|
64 |
-
|
65 |
-
|
66 |
-
|
67 |
-
|
68 |
-
|
69 |
-
|
70 |
-
|
71 |
-
|
72 |
-
|
73 |
-
|
74 |
-
|
75 |
-
|
76 |
-
|
77 |
-
|
78 |
-
|
79 |
-
|
80 |
-
|
81 |
-
|
82 |
-
[More Information Needed]
|
83 |
-
|
84 |
-
### Training Procedure
|
85 |
-
|
86 |
-
<!-- This relates heavily to the Technical Specifications. Content here should link to that section when it is relevant to the training procedure. -->
|
87 |
-
|
88 |
-
#### Preprocessing [optional]
|
89 |
-
|
90 |
-
[More Information Needed]
|
91 |
-
|
92 |
-
|
93 |
-
#### Training Hyperparameters
|
94 |
-
|
95 |
-
- **Training regime:** [More Information Needed] <!--fp32, fp16 mixed precision, bf16 mixed precision, bf16 non-mixed precision, fp16 non-mixed precision, fp8 mixed precision -->
|
96 |
-
|
97 |
-
#### Speeds, Sizes, Times [optional]
|
98 |
-
|
99 |
-
<!-- This section provides information about throughput, start/end time, checkpoint size if relevant, etc. -->
|
100 |
-
|
101 |
-
[More Information Needed]
|
102 |
-
|
103 |
-
## Evaluation
|
104 |
-
|
105 |
-
<!-- This section describes the evaluation protocols and provides the results. -->
|
106 |
-
|
107 |
-
### Testing Data, Factors & Metrics
|
108 |
-
|
109 |
-
#### Testing Data
|
110 |
-
|
111 |
-
<!-- This should link to a Dataset Card if possible. -->
|
112 |
-
|
113 |
-
[More Information Needed]
|
114 |
-
|
115 |
-
#### Factors
|
116 |
-
|
117 |
-
<!-- These are the things the evaluation is disaggregating by, e.g., subpopulations or domains. -->
|
118 |
-
|
119 |
-
[More Information Needed]
|
120 |
-
|
121 |
-
#### Metrics
|
122 |
-
|
123 |
-
<!-- These are the evaluation metrics being used, ideally with a description of why. -->
|
124 |
-
|
125 |
-
[More Information Needed]
|
126 |
-
|
127 |
-
### Results
|
128 |
-
|
129 |
-
[More Information Needed]
|
130 |
-
|
131 |
-
#### Summary
|
132 |
-
|
133 |
-
|
134 |
-
|
135 |
-
## Model Examination [optional]
|
136 |
-
|
137 |
-
<!-- Relevant interpretability work for the model goes here -->
|
138 |
-
|
139 |
-
[More Information Needed]
|
140 |
-
|
141 |
-
## Environmental Impact
|
142 |
-
|
143 |
-
<!-- Total emissions (in grams of CO2eq) and additional considerations, such as electricity usage, go here. Edit the suggested text below accordingly -->
|
144 |
-
|
145 |
-
Carbon emissions can be estimated using the [Machine Learning Impact calculator](https://mlco2.github.io/impact#compute) presented in [Lacoste et al. (2019)](https://arxiv.org/abs/1910.09700).
|
146 |
-
|
147 |
-
- **Hardware Type:** [More Information Needed]
|
148 |
-
- **Hours used:** [More Information Needed]
|
149 |
-
- **Cloud Provider:** [More Information Needed]
|
150 |
-
- **Compute Region:** [More Information Needed]
|
151 |
-
- **Carbon Emitted:** [More Information Needed]
|
152 |
-
|
153 |
-
## Technical Specifications [optional]
|
154 |
-
|
155 |
-
### Model Architecture and Objective
|
156 |
-
|
157 |
-
[More Information Needed]
|
158 |
-
|
159 |
-
### Compute Infrastructure
|
160 |
-
|
161 |
-
[More Information Needed]
|
162 |
-
|
163 |
-
#### Hardware
|
164 |
-
|
165 |
-
[More Information Needed]
|
166 |
-
|
167 |
-
#### Software
|
168 |
-
|
169 |
-
[More Information Needed]
|
170 |
-
|
171 |
-
## Citation [optional]
|
172 |
-
|
173 |
-
<!-- If there is a paper or blog post introducing the model, the APA and Bibtex information for that should go in this section. -->
|
174 |
-
|
175 |
-
**BibTeX:**
|
176 |
-
|
177 |
-
[More Information Needed]
|
178 |
-
|
179 |
-
**APA:**
|
180 |
-
|
181 |
-
[More Information Needed]
|
182 |
-
|
183 |
-
## Glossary [optional]
|
184 |
-
|
185 |
-
<!-- If relevant, include terms and calculations in this section that can help readers understand the model or model card. -->
|
186 |
-
|
187 |
-
[More Information Needed]
|
188 |
-
|
189 |
-
## More Information [optional]
|
190 |
-
|
191 |
-
[More Information Needed]
|
192 |
-
|
193 |
-
## Model Card Authors [optional]
|
194 |
-
|
195 |
-
[More Information Needed]
|
196 |
-
|
197 |
-
## Model Card Contact
|
198 |
-
|
199 |
-
[More Information Needed]
|
|
|
1 |
---
|
2 |
+
license: mit
|
3 |
+
datasets:
|
4 |
+
- TIGER-Lab/AceCode-89K
|
5 |
+
language:
|
6 |
+
- en
|
7 |
+
base_model:
|
8 |
+
- Qwen/Qwen2.5-Coder-7B-Instruct
|
9 |
+
tags:
|
10 |
+
- acecoder
|
11 |
+
- code
|
12 |
+
- Qwen
|
13 |
---
|
14 |
|
|
|
15 |
|
16 |
+
# 🂡 AceCode-89K
|
17 |
+
|
18 |
+
[Paper](#) |
|
19 |
+
[Github](https://github.com/TIGER-AI-Lab/AceCoder) |
|
20 |
+
[AceCode-89K](https://huggingface.co/datasets/TIGER-Lab/AceCode-89K) |
|
21 |
+
[AceCodePair-300K](https://huggingface.co/datasets/TIGER-Lab/AceCodePair-300K) |
|
22 |
+
[RM/RL Models](https://huggingface.co/collections/TIGER-Lab/acecoder-67a16011a6c7d65cad529eba)
|
23 |
+
|
24 |
+
|
25 |
+
We introduce AceCoder, the first work to propose a fully automated pipeline for synthesizing large-scale reliable tests used for the reward model training and reinforcement learning in the coding scenario. To do this, we curated the dataset AceCode-89K, where we start from a seed code dataset and prompt powerful LLMs to "imagine" proper test cases for the coding question and filter the noisy ones. We sample inferences from existing coder models and compute their pass rate as the reliable and verifiable rewards for both training the reward model and conducting the reinforcement learning for coder LLM.
|
26 |
+
|
27 |
+
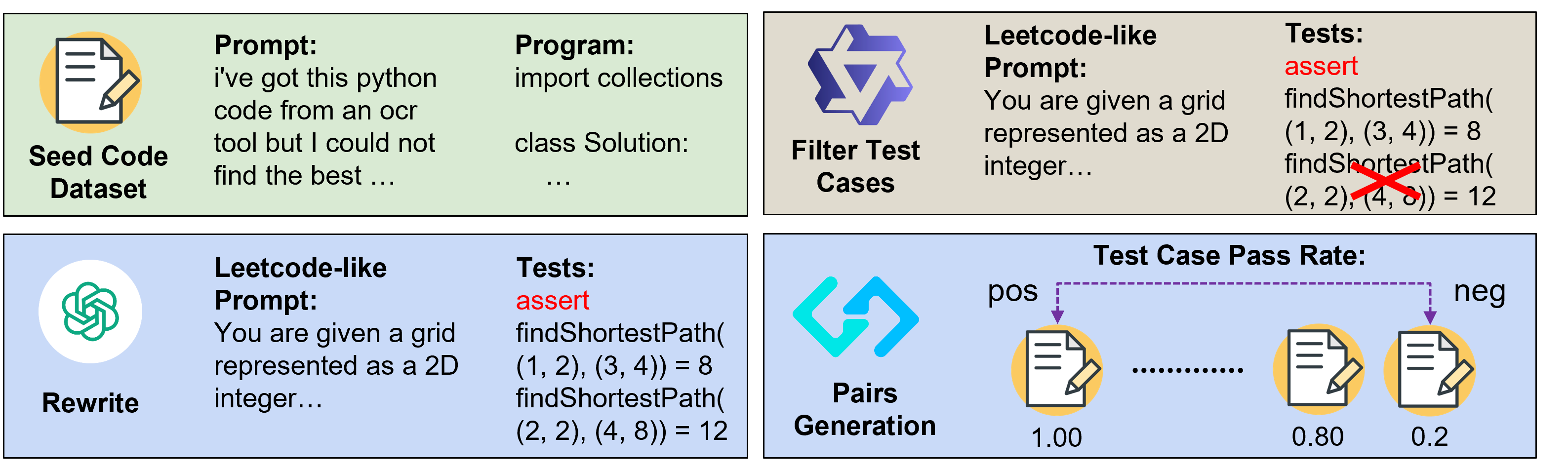
|
28 |
+
|
29 |
+
|
30 |
+
## Note
|
31 |
+
- **This model official is trained on the hard version of [TIGER-Lab/AceCode-89K](https://huggingface.co/datasets/TIGER-Lab/AceCode-89K) with about 22k examples, using the binary pass rate (rule based reward) as the reward**
|
32 |
+
<!-- - **This model official is trained on the hard version of [TIGER-Lab/AceCode-89K](https://huggingface.co/datasets/TIGER-Lab/AceCode-89K) with about 22k examples, using the [TIGER-Lab/AceCodeRM-7B](https://huggingface.co/TIGER-Lab/AceCodeRM-7B) as the reward** -->
|
33 |
+
- You can reproduce the hard version of [TIGER-Lab/AceCode-89K](https://huggingface.co/datasets/TIGER-Lab/AceCode-89K) using [script in our Github](#)
|
34 |
+
- The training takes 6 hours to finish on 8 x H100 GPUs in around 80 optimization steps.
|
35 |
+
- To reproduce the training, please refer to our [training script in the Github](#)
|
36 |
+
- To use the model, please refer to the codes in [Qwen/Qwen2.5-7B-Instruct](https://huggingface.co/Qwen/Qwen2.5-7B-Instruct)
|
37 |
+
- Training [wandb link](https://wandb.ai/dongfu/openrlhf_train_ppo/runs/5xqjy4uu)
|
38 |
+
|
39 |
+
## Usage
|
40 |
+
```python
|
41 |
+
from transformers import AutoModelForCausalLM, AutoTokenizer
|
42 |
+
|
43 |
+
model_name = "TIGER-Lab/AceCoder-Qwen2.5-Coder-7B-Ins-Rule"
|
44 |
+
|
45 |
+
model = AutoModelForCausalLM.from_pretrained(
|
46 |
+
model_name,
|
47 |
+
torch_dtype="auto",
|
48 |
+
device_map="auto"
|
49 |
+
)
|
50 |
+
tokenizer = AutoTokenizer.from_pretrained(model_name)
|
51 |
+
|
52 |
+
prompt = "Give me a short introduction to large language model."
|
53 |
+
messages = [
|
54 |
+
{"role": "system", "content": "You are Qwen, created by Alibaba Cloud. You are a helpful assistant."},
|
55 |
+
{"role": "user", "content": prompt}
|
56 |
+
]
|
57 |
+
text = tokenizer.apply_chat_template(
|
58 |
+
messages,
|
59 |
+
tokenize=False,
|
60 |
+
add_generation_prompt=True
|
61 |
+
)
|
62 |
+
model_inputs = tokenizer([text], return_tensors="pt").to(model.device)
|
63 |
+
|
64 |
+
generated_ids = model.generate(
|
65 |
+
**model_inputs,
|
66 |
+
max_new_tokens=512
|
67 |
+
)
|
68 |
+
generated_ids = [
|
69 |
+
output_ids[len(input_ids):] for input_ids, output_ids in zip(model_inputs.input_ids, generated_ids)
|
70 |
+
]
|
71 |
+
|
72 |
+
response = tokenizer.batch_decode(generated_ids, skip_special_tokens=True)[0]
|
73 |
+
```
|
74 |
+
|
75 |
+
## Performance
|
76 |
+
|
77 |
+
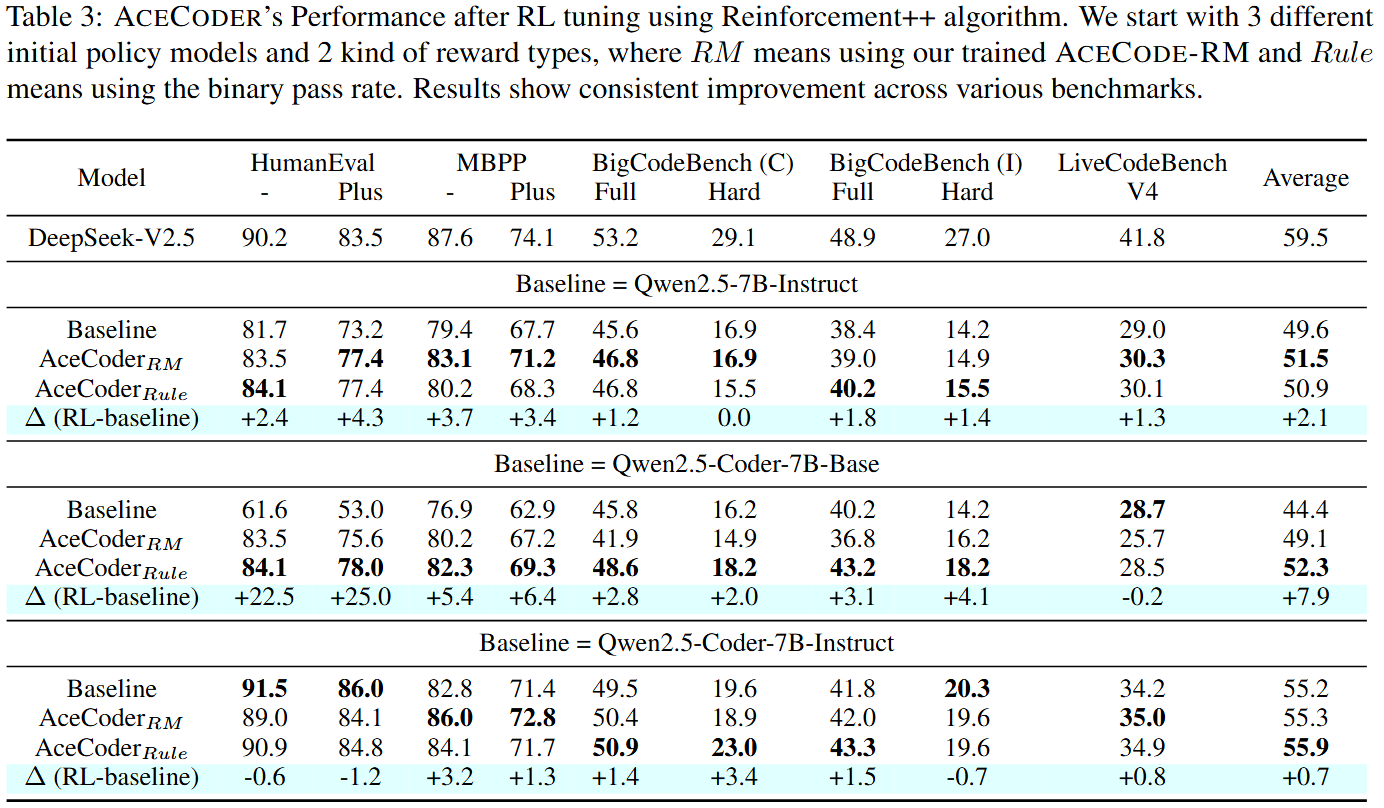
|
78 |
+
|
79 |
+
## Citation
|
80 |
+
```bibtex
|
81 |
+
@article{AceCoder,
|
82 |
+
title={AceCoder: Acing Coder RL via Automated Test-Case Synthesis},
|
83 |
+
author={Zeng, Huaye and Jiang, Dongfu and Wang, Haozhe and Nie, Ping and Chen, Xiaotong and Chen, Wenhu},
|
84 |
+
journal={ArXiv},
|
85 |
+
year={2025},
|
86 |
+
volume={abs/2207.01780}
|
87 |
+
}
|
88 |
+
```
|
|
|
|
|
|
|
|
|
|
|
|
|
|
|
|
|
|
|
|
|
|
|
|
|
|
|
|
|
|
|
|
|
|
|
|
|
|
|
|
|
|
|
|
|
|
|
|
|
|
|
|
|
|
|
|
|
|
|
|
|
|
|
|
|
|
|
|
|
|
|
|
|
|
|
|
|
|
|
|
|
|
|
|
|
|
|
|
|
|
|
|
|
|
|
|
|
|
|
|
|
|
|
|
|
|
|
|
|
|
|
|
|
|
|
|
|
|
|
|
|
|
|
|
|
|
|
|
|
|
|
|
|
|
|
|
|
|
|
|
|
|
|
|
|
|
|
|
|
|
|
|
|
|
|
|
|
|
|
|
|
|
|
|
|
|
|
|
|
|
|
|
|
|
|
|
|
|
|
|
|
|
|
|
|
|
|
|
|
|
|
|
|
|
|
|
|
|
|
|
|
|
|
|
|
|
|
|
|
|
|
|
|
|
|
|
|
|
|
|
|
|
|
|
|
|
|
|
|
|
|
|
|
|
|
|
|
|